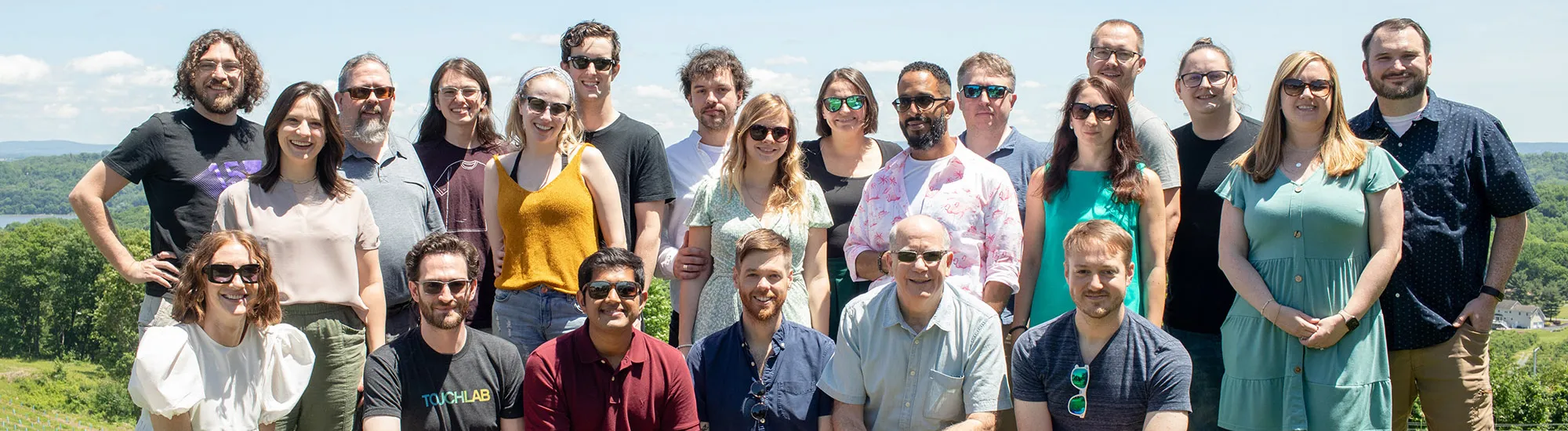
About Touchlab
Open positions
Touchlab is looking for an iOS developer with Kotlin experience who wants to work with us and have a significant impact on the development and maturity of the Kotlin Multiplatform (KMP) ecosystem.
Remote
Touchlab is looking for a Kotlin developer with significant Kotlin Multiplatform experience who wants to work with us and have a major impact on the development and maturity of the Kotlin Multiplatform ecosystem.
Remote - Brazil
We're expanding our contractor pool and seeking multiple talented developers to work with us on a variety of innovative projects.
Remote
Touchlab is looking for a Technical Project Manager. We are the global experts in Kotlin Multiplatform (KMP) for Mobile tech. As KMP adoption continues to grow, we need to execute new and exciting projects for external clients and internal R&D.
Remote
Touchlab Leadership
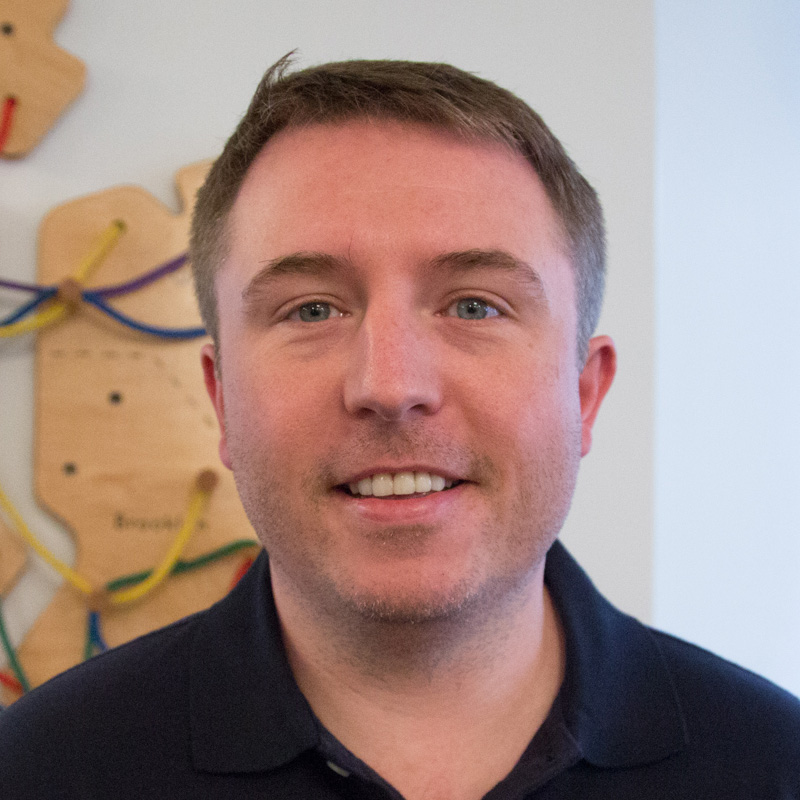
Kevin Galligan
Technical Partner
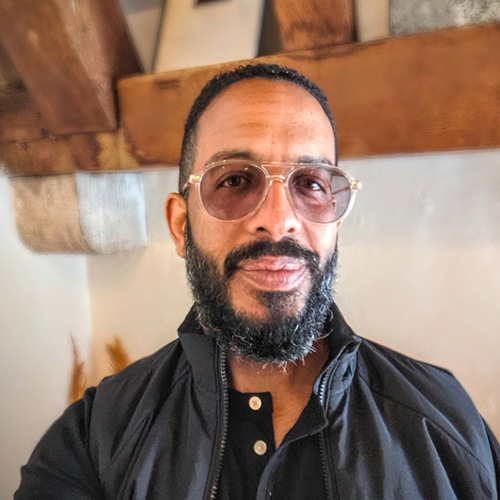
Jeff Namnum
Managing Partner
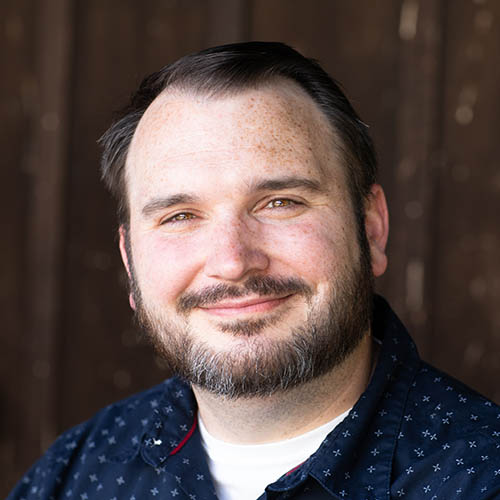
Sam Hill
Engineering Manager
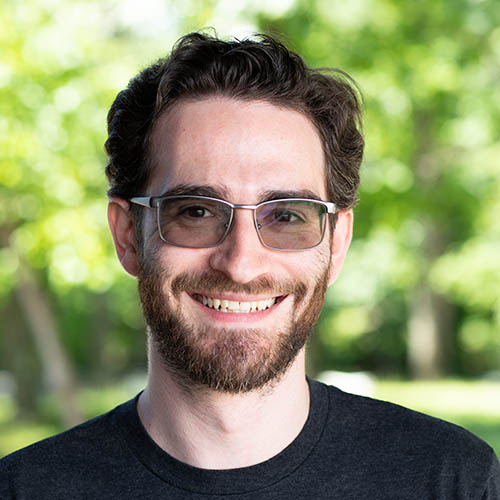
Justin Mancinelli
Dir. Product Strategy
Trusted by industry leaders
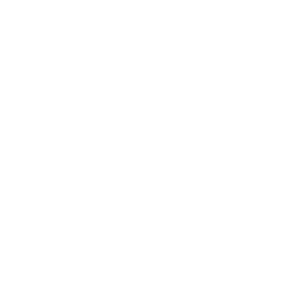
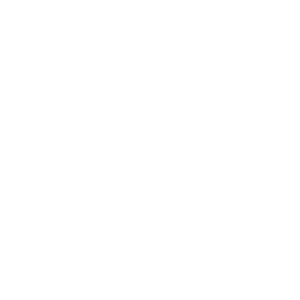
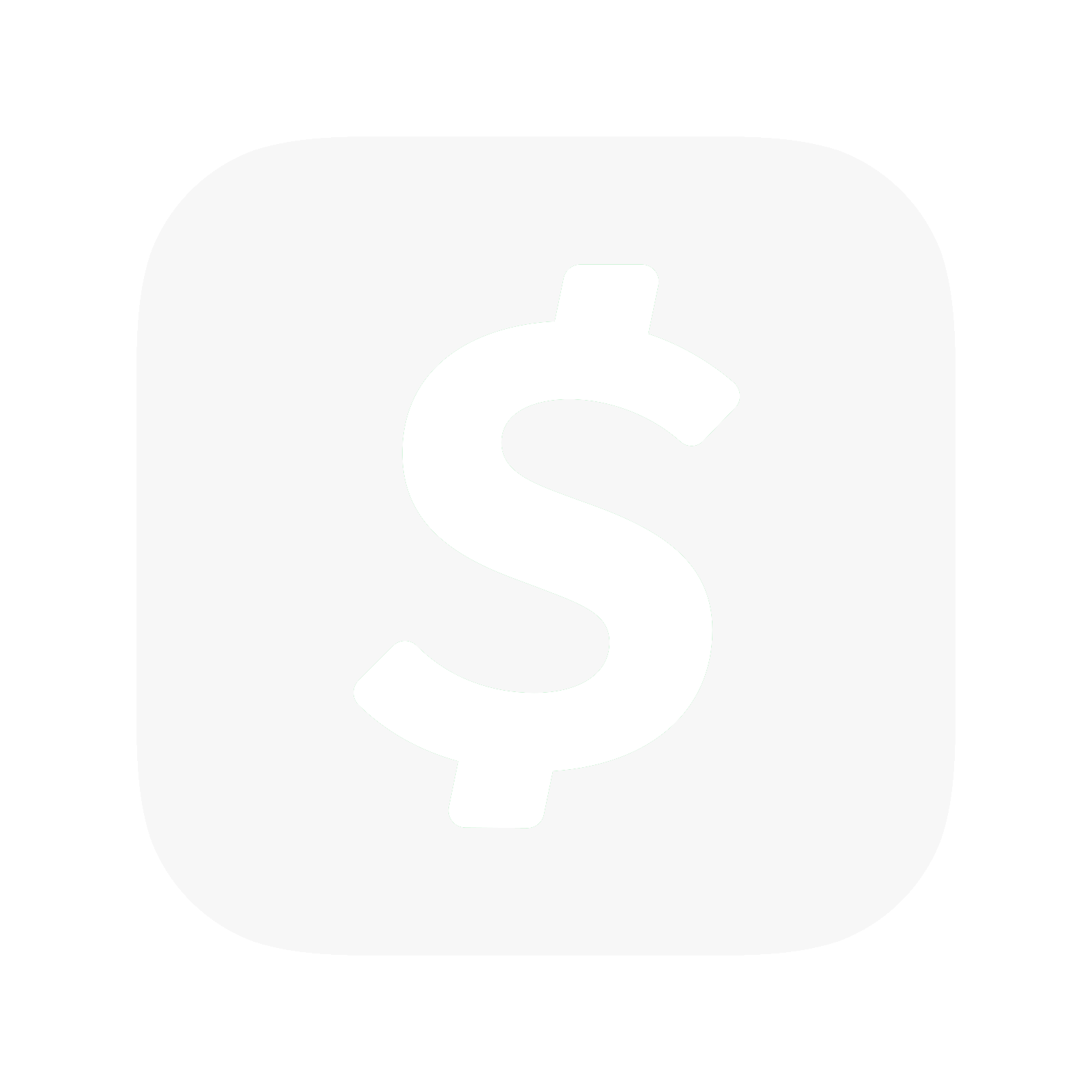
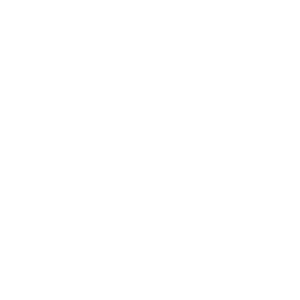
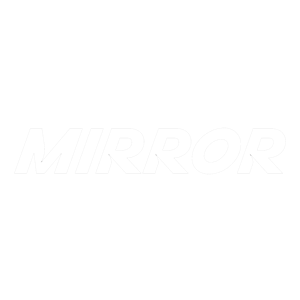
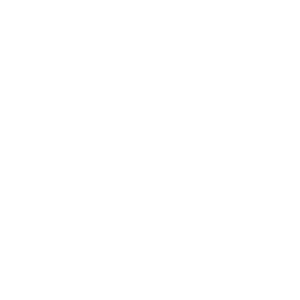
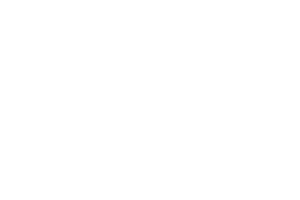
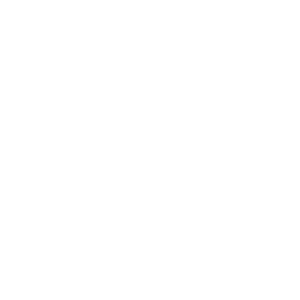